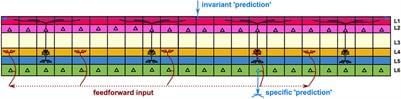
The abstract basis of modern computation is the formal description of a finite state machine, the Universal Turing Machine, based on manipulation of integers and logic symbols. In this contribution to the discourse on the computer-brain analogy, we discuss the extent to which analog computing, as performed by the mammalian brain, is like and unlike the digital computing of Universal Turing Machines. We begin with ordinary reality being a permanent dialog between continuous and discontinuous worlds. So it is with computing, which can be analog or digital, and is often mixed. The theory behind computers is essentially digital, but efficient simulations of phenomena can be performed by analog devices; indeed, any physical calculation requires implementation in the physical world and is therefore analog to some extent, despite being based on abstract logic and arithmetic. The mammalian brain, comprised of neuronal networks, functions as an analog device and has given rise to artificial neural networks that are implemented as digital algorithms but function as analog models would. Analog constructs compute with the implementation of a variety of feedback and feedforward loops. In contrast, digital algorithms allow the implementation of recursive processes that enable them to generate unparalleled emergent properties. We briefly illustrate how the cortical organization of neurons can integrate signals and make predictions analogically. While we conclude that brains are not digital computers, we speculate on the recent implementation of human writing in the brain as a possible digital path that slowly evolves the brain into a genuine (slow) Turing machine.
The present essay explores key similarities and differences in the process of computation by the brains of animals and by digital computing, by anchoring the exploration on the essential properties of a Universal Turning Machine, the abstract foundation of modern digital computing. In this context, we try to explicitly distance XVIIIth century mechanical automata from modern machines, understanding that when computation allows recursion, it changes the consequences of determinism. A mechanical device is usually both deterministic and predictable, while computation involving recursion is deterministic but not necessarily predictable. For example, while it is possible to design an algorithm that computes the decimal digits of π, the value of any finite sequence following the nth digit, cannot (yet) be computed, hence predicted, with n sufficiently large.