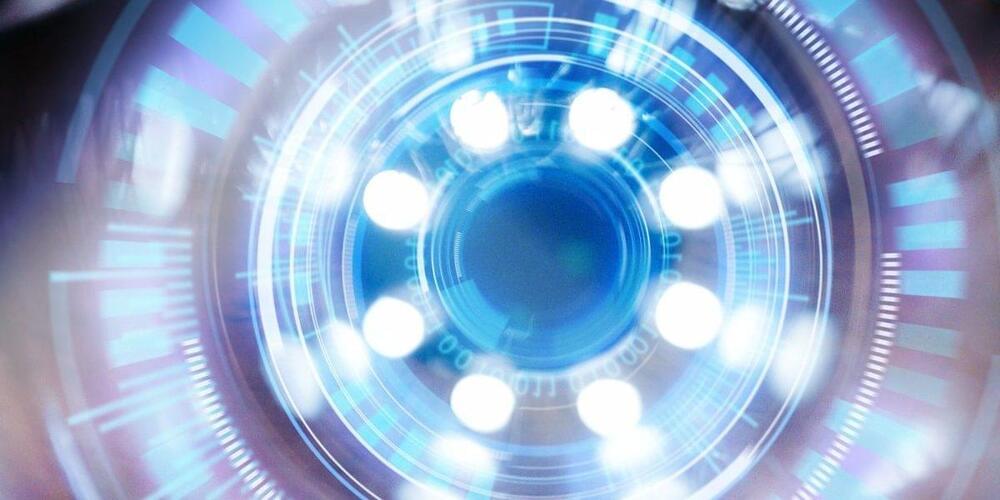
According to new research by Datagen, 99% of computer vision (CV) teams have had a machine learning (ML) project canceled due to insufficient training data. Delays, meanwhile, appear truly ubiquitous, with 100% of teams reporting experiencing significant project delays due to insufficient training data. The research also indicates that these training data challenges come in many forms and affect CV teams in near-equal measure. The top issues experienced by CV teams include poor annotation (48%), inadequate domain coverage (47%), and simple scarcity (44%).
The scarcity of robust, domain-specific training data is only compounded by the fact that the field of computer vision is lacking many well-defined standards or best practices. When asked how training data is typically gathered at their organizations, respondents revealed a patchwork of sources and methodologies are being employed both across the field and within individual organizations. Whether synthetic or real, collected in-house or sourced from public datasets, organizations appear to be utilizing any and all data they can in order to train their computer vision models.
However, computer vision teams have already identified and begun to embrace synthetic data as a solution. Ninety-six percent of CV teams reported having already adopted the use of synthetic data to help train their AI/ML models. Nevertheless, the quality, source, and proportion of synthetic data that’s used remains highly variable across the field, and only 6% of teams currently use synthetic data exclusively.