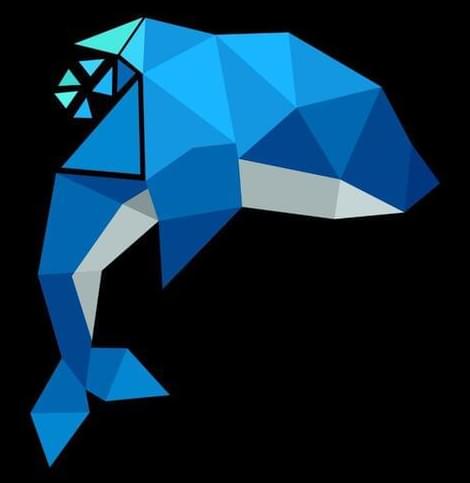
The remarkable zero-shot learning capabilities demonstrated by large foundation models (LFMs) like ChatGPT and GPT-4 have sparked a question: Can these models autonomously supervise their behavior or other models with minimal human intervention? To explore this, a team of Microsoft researchers introduces Orca, a 13-billion parameter model that learns complex explanation traces and step-by-step thought processes from GPT-4. This innovative approach significantly improves the performance of existing state-of-the-art instruction-tuned models, addressing challenges related to task diversity, query complexity, and data scaling.
The researchers acknowledge that the query and response pairs from GPT-4 can provide valuable guidance for student models. Therefore, they enhance these pairs by adding detailed responses that offer a better understanding of the reasoning process employed by the teachers when generating their responses. By incorporating these explanation traces, Orca equips student models with improved reasoning and comprehension skills, effectively bridging the gap between teachers and students.
The research team utilizes the Flan 2022 Collection to enhance Orca’s learning process further. The team samples tasks from this extensive collection to ensure a diverse mix of challenges. These tasks are then sub-sampled to generate complex prompts, which serve as queries for LFMs. This approach creates a diverse and rich training set that facilitates robust learning for the Orca, enabling it to tackle a wide range of tasks effectively.