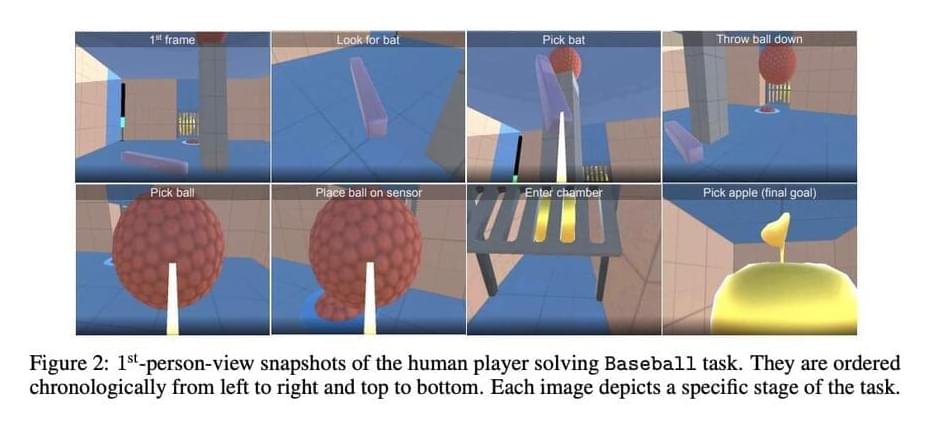
DeepMind Researchers Develop ‘BYOL-Explore’, A Curiosity-Driven Exploration Algorithm That Harnesses The Power Of Self-Supervised Learning To Solve Sparse-Reward Partially-Observable Tasks
Reinforcement learning (RL) requires exploration of the environment. Exploration is even more critical when extrinsic incentives are few or difficult to obtain. Due to the massive size of the environment, it is impractical to visit every location in rich settings due to the range of helpful exploration paths. Consequently, the question is: how can an agent decide which areas of the environment are worth exploring? Curiosity-driven exploration is a viable approach to tackle this problem. It entails learning a world model, a predictive model of specific knowledge about the world, and (ii) exploiting disparities between the world model’s predictions and experience to create intrinsic rewards.
An RL agent that maximizes these intrinsic incentives steers itself toward situations where the world model is unreliable or unsatisfactory, creating new paths for the world model. In other words, the quality of the exploration policy is influenced by the characteristics of the world model, which in turn helps the world model by collecting new data. Therefore, it might be crucial to approach learning the world model and learning the exploratory policy as one cohesive problem to be solved rather than two separate tasks. Deepmind researchers keeping this in mind, introduced a curiosity-driven exploration algorithm BYOL-Explore. Its attraction stems from its conceptual simplicity, generality, and excellent performance.
The strategy is based on Bootstrap Your Own Latent (BYOL), a self-supervised latent-predictive method that forecasts an earlier version of its latent representation. In order to handle the problems of creating the representation of the world model and the curiosity-driven policy, BYOL-Explore learns a world model with a self-supervised prediction loss and trains a curiosity-driven policy using the same loss. Computer vision, learning about graph representations, and RL representation learning have all successfully used this bootstrapping approach. In contrast, BYOL-Explore goes one step further and not only learns a flexible world model but also exploits the world model’s loss to motivate exploration.