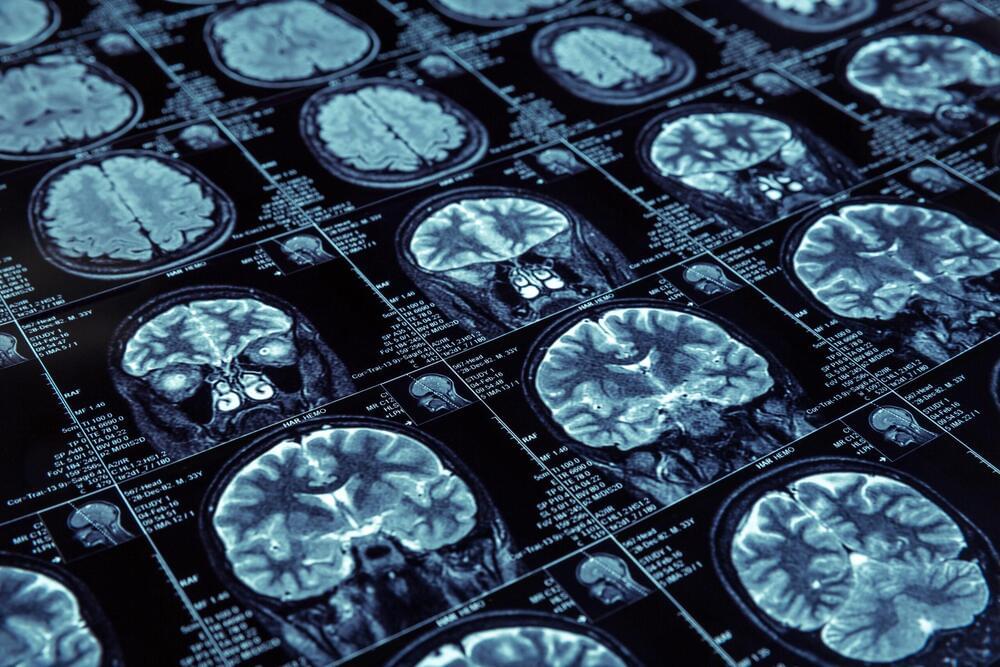
A new study, published in “Nature Communications” this week, led by Jake Gavenas PhD, while he was a PhD student at the Brain Institute at Chapman University, and co-authored by two faculty members of the Brain Institute, Uri Maoz and Aaron Schurger, examines how the brain initiates spontaneous actions. In addition to demonstrating how spontaneous action emerges without environmental input, this study has implications for the origins of slow ramping of neural activity before movement onset—a commonly-observed but poorly understood phenomenon.
In their study, Gavenas and colleagues propose an answer to that question. They simulated spontaneous activity in simple neural networks and compared this simulated activity to intracortical recordings of humans when they moved spontaneously. The study results suggest something striking: many rapidly fluctuating neurons can interact in a network to give rise to very slow fluctuations at the level of the population.
Imagine, for example, standing atop a high-dive platform and trying to summon the willpower to jump. Nothing in the outside world tells you when to jump; that decision comes from within. At some point you experience deciding to jump and then you jump. In the background, your brain (or, more specifically, your motor cortex) sends electrical signals that cause carefully coordinated muscle contractions across your body, resulting in you running and jumping. But where in the brain do these signals originate, and how do they relate to the conscious experience of willing your body to move?