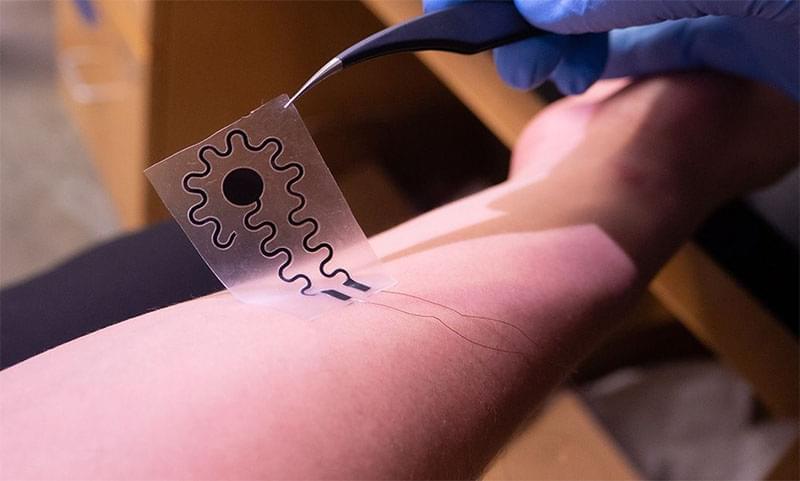
A team of researchers led by the University of California San Diego has developed a soft, stretchy electronic device capable of simulating the feeling of pressure or vibration when worn on the skin. This device, reported in a paper published in Science Robotics (“Conductive block copolymer elastomers and psychophysical thresholding for accurate haptic effects”), represents a step towards creating haptic technologies that can reproduce a more varied and realistic range of touch sensations.
The device consists of a soft, stretchable electrode attached to a silicone patch. It can be worn like a sticker on either the fingertip or forearm. The electrode, in direct contact with the skin, is connected to an external power source via wires. By sending a mild electrical current through the skin, the device can produce sensations of either pressure or vibration depending on the signal’s frequency.
Soft, stretchable electrode recreates sensations of vibration or pressure on the skin through electrical stimulation. (Image: Liezel Labios, UC San Diego Jacobs School of Engineering)