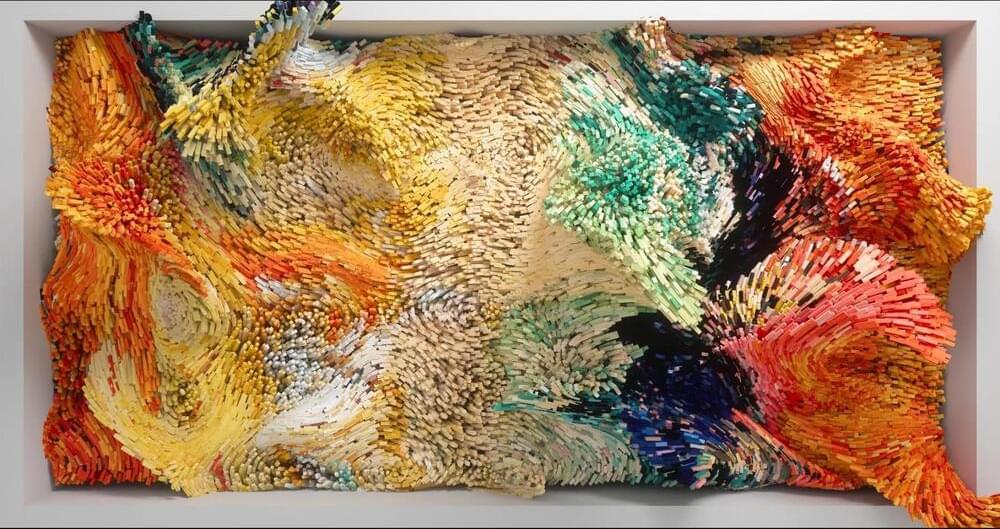
Aaron Vick is a multi-x founder, former CEO, best-selling author, process and workflow nerd and early-stage/growth advisor focused on Web3.
The age of artificial intelligence (AI) is transforming the landscape of creativity, challenging our understanding of creator rights and digital identity. As AI becomes an integral part of the creative process, collaborating with human minds to push the boundaries of imagination and innovation, we find ourselves in a new era that demands reevaluating the essence of authorship.
This AI renaissance is not just about the tools we use to create; it is about the fundamental shift in how we perceive and value creativity. In a world where AI can generate art, music and literature that rivals the works of human creators, we must reconsider what it means to be an author, an artist or a creator. The lines between human and machine creativity are blurring, giving rise to new forms of expression and collaboration that were once unimaginable.