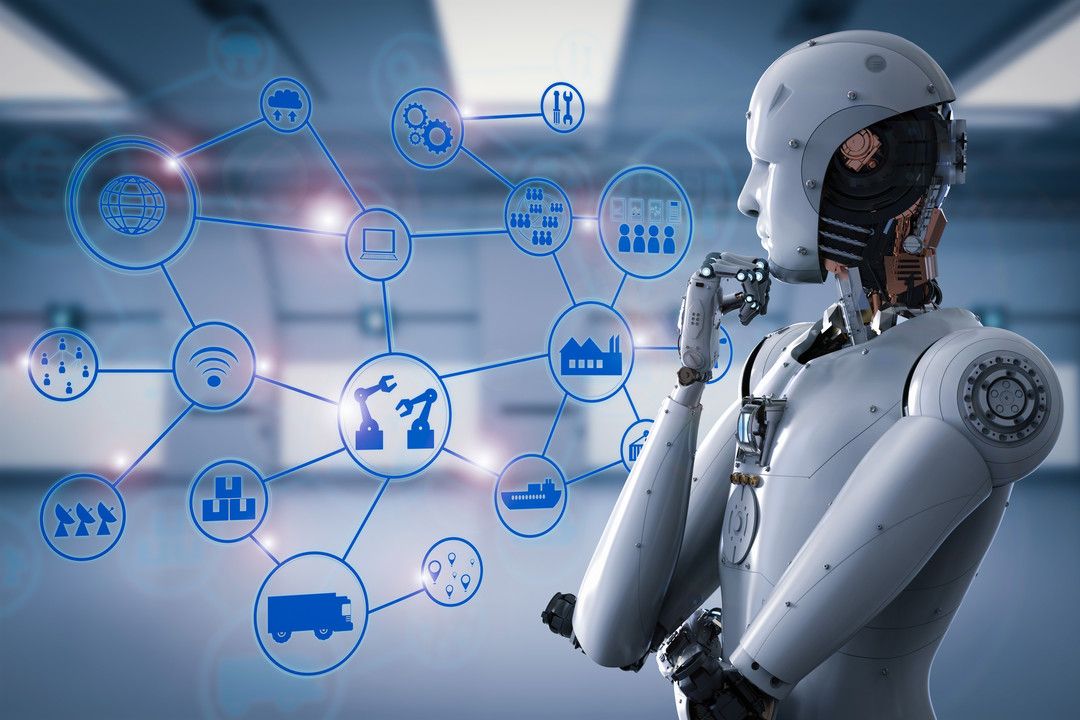
So much talk about AI and robots taking our jobs. Well, guess what, it’s already happening and the rate of change will only increase. I estimate that about 5% of jobs have been automated — both blue collar manufacturing jobs, as well as, this time, low-level white collar jobs — think back office, paralegals, etc. There’s a thing called RPA, or Robot Process Automation, which is hollowing out back office jobs at an alarming rate, using rules based algorithms and expert systems. This will rapidly change with the introduction of deep learning algorithms into these “robot automation” systems, making them intelligent, capable of making intuitive decisions and therefore replacing more highly skilled and creative jobs. So if we’re on an exponential curve, and we’ve managed to automate around 5% of jobs in the past six years, say, and the doubling is every two years, that means by 2030, almost all jobs will be automated. Remember, the exponential math means 1, 2, 4, 8, 16, 32, 64, 100%, with the doubling every two years.
We are definitely going to need a basic income to prevent people (doctors, lawyers, drivers, teachers, scientists, manufacturers, craftsmen) from going homeless once their jobs are automated away. This will need to be worked out at the government level — the sooner the better, because exponentials have a habit of creeping up on people and then surprising society with the intensity and rapidity of the disruptive change they bring. I’m confident that humanity can and will rise to the challenges ahead, and it is well to remember that economics is driven by technology, not the other way around. Education, as usual, is definitely the key to meeting these challenges head on and in a fully informed way. My only concern is when governments will actually start taking this situation seriously enough to start taking bold action. There certainly is no time like the present.
Read more