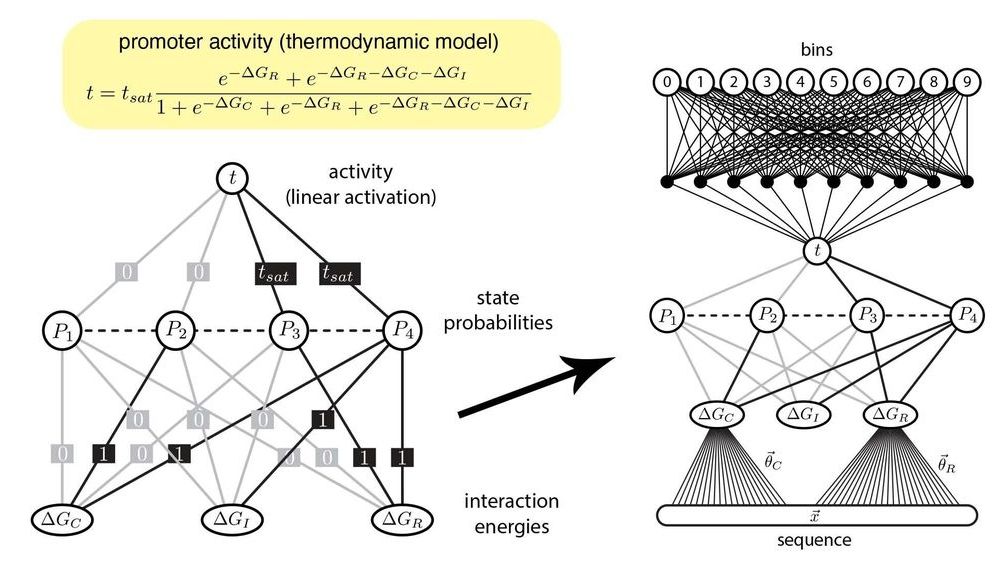
In this age of “big data,” artificial intelligence (AI) has become a valuable ally for scientists. Machine learning algorithms, for instance, are helping biologists make sense of the dizzying number of molecular signals that control how genes function. But as new algorithms are developed to analyze even more data, they also become more complex and more difficult to interpret. Quantitative biologists Justin B. Kinney and Ammar Tareen have a strategy to design advanced machine learning algorithms that are easier for biologists to understand.
The algorithms are a type of artificial neural network (ANN). Inspired by the way neurons connect and branch in the brain, ANNs are the computational foundations for advanced machine learning. And despite their name, ANNs are not exclusively used to study brains.
Biologists, like Tareen and Kinney, use ANNs to analyze data from an experimental method called a “massively parallel reporter assay” (MPRA) which investigates DNA. Using this data, quantitative biologists can make ANNs that predict which molecules control specific genes in a process called gene regulation.