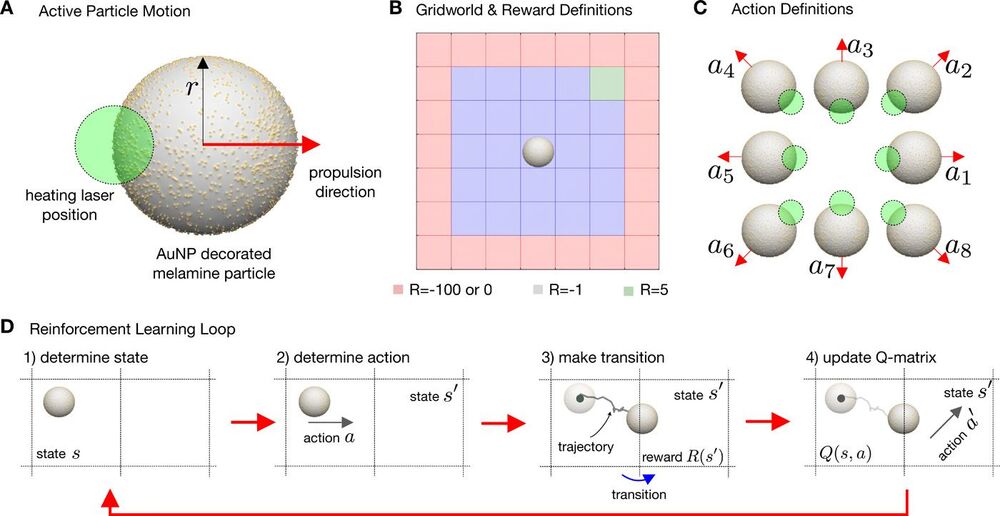
Artificial microswimmers that can replicate the complex behavior of active matter are often designed to mimic the self-propulsion of microscopic living organisms. However, compared with their living counterparts, artificial microswimmers have a limited ability to adapt to environmental signals or to retain a physical memory to yield optimized emergent behavior. Different from macroscopic living systems and robots, both microscopic living organisms and artificial microswimmers are subject to Brownian motion, which randomizes their position and propulsion direction. Here, we combine real-world artificial active particles with machine learning algorithms to explore their adaptive behavior in a noisy environment with reinforcement learning. We use a real-time control of self-thermophoretic active particles to demonstrate the solution of a simple standard navigation problem under the inevitable influence of Brownian motion at these length scales. We show that, with external control, collective learning is possible. Concerning the learning under noise, we find that noise decreases the learning speed, modifies the optimal behavior, and also increases the strength of the decisions made. As a consequence of time delay in the feedback loop controlling the particles, an optimum velocity, reminiscent of optimal run-and-tumble times of bacteria, is found for the system, which is conjectured to be a universal property of systems exhibiting delayed response in a noisy environment.
Living organisms adapt their behavior according to their environment to achieve a particular goal. Information about the state of the environment is sensed, processed, and encoded in biochemical processes in the organism to provide appropriate actions or properties. These learning or adaptive processes occur within the lifetime of a generation, over multiple generations, or over evolutionarily relevant time scales. They lead to specific behaviors of individuals and collectives. Swarms of fish or flocks of birds have developed collective strategies adapted to the existence of predators (1), and collective hunting may represent a more efficient foraging tactic (2). Birds learn how to use convective air flows (3). Sperm have evolved complex swimming patterns to explore chemical gradients in chemotaxis (4), and bacteria express specific shapes to follow gravity (5).
Inspired by these optimization processes, learning strategies that reduce the complexity of the physical and chemical processes in living matter to a mathematical procedure have been developed. Many of these learning strategies have been implemented into robotic systems (7–9). One particular framework is reinforcement learning (RL), in which an agent gains experience by interacting with its environment (10). The value of this experience relates to rewards (or penalties) connected to the states that the agent can occupy. The learning process then maximizes the cumulative reward for a chain of actions to obtain the so-called policy. This policy advises the agent which action to take. Recent computational studies, for example, reveal that RL can provide optimal strategies for the navigation of active particles through flows (11–13), the swarming of robots (14–16), the soaring of birds , or the development of collective motion (17).