Knowing a little about the local connections on flight maps and other networks can reveal a lot about a system’s global structure.
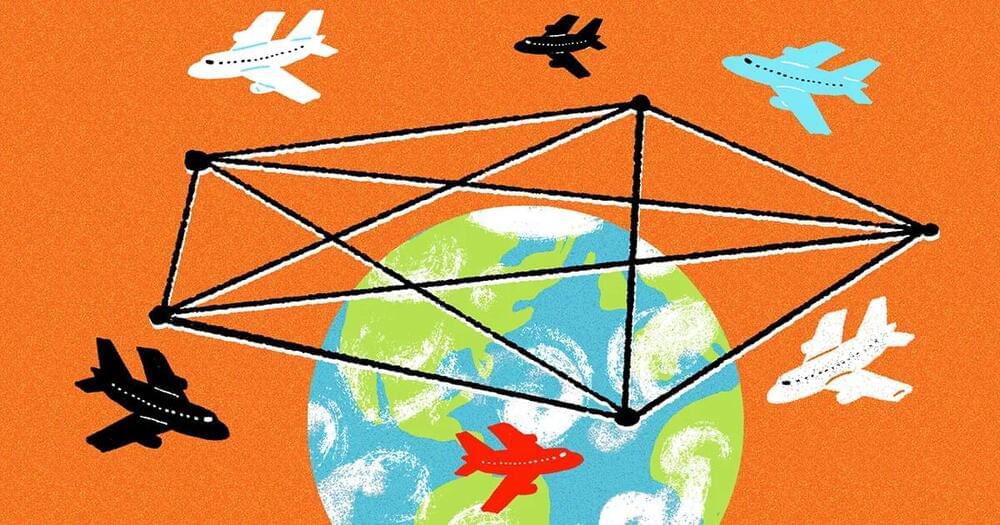
The digital devices that we rely on so heavily in our day-to-day and professional lives today—smartphones, tablets, laptops, fitness trackers, etc.—use traditional computational technology. Traditional computers rely on a series of mathematical equations that use electrical impulses to encode information in a binary system of 1s and 0s. This information is transmitted through quantitative measurements called “bits.”
Unlike traditional computing, quantum computing relies on the principles of quantum theory, which address principles of matter and energy on an atomic and subatomic scale. With quantum computing, equations are no longer limited to 1s and 0s, but instead can transmit information in which particles exist in both states, the 1 and the 0, at the same time.
Quantum computing measures electrons or photons. These subatomic particles are known as quantum bits, or ” qubits.” The more qubits are used in a computational exercise, the more exponentially powerful the scope of the computation can be. Quantum computing has the potential to solve equations in a matter of minutes that would take traditional computers tens of thousands of years to work out.
Everyone knows that 2 + 2 = 4, but why do we have arithmetic in the first place, and why is it true? Researchers at the University of Canterbury have recently answered these questions by “reverse engineering” arithmetic from a psychological perspective. To do this, they considered all possible ways that quantities could be combined, and proved (for the first time in mathematical terms) that addition and multiplication are the simplest.
Their proof is based on four assumptions —principles of perceptual organization—that shape how we and other animals experience the world. These assumptions eliminate all possibilities except arithmetic, like how a sculptor’s work reveals a statue hidden in a block of stone.
Monotonicity is the idea of “things changing in the same direction,” and helps us keep track of our place in the world, so that when we approach an object it looms larger but smaller when we move away. Convexity is grounded in intuitions of betweenness. For example, the four corners of a football pitch define the playing field even without boundary lines connecting them. Continuity describes the smoothness with which objects seem to move in space and time. Isomorphism is the idea of sameness or analogy. It’s what allows us to recognize that a cat is more similar to a dog than it is to a rock.
Originally developed nearly a century ago by physicists studying neutron diffusion, Monte Carlo simulations are mathematical models that use random numbers to simulate different kinds of events. As a simple example of how they work, imagine you have a pair of six-sided dice, and you’d like to determine the probability of the dice landing on any given number.
“You take your dice, and you repeat the same exercise of throwing them on the table, and you look at the outcome,” says Susanna Guatelli, associate professor of physics at the University of Wollongong in Australia.
By repeating the dice-throwing experiment and recording the number of times your dice land on each number, you can build a “probability distribution”—a list giving you the likelihood your dice will land on each possible outcome.
In today’s column, I am going to identify and explain the momentous pairing of both generative AI and data science. These two realms are each monumental in their own respective ways, thus they are worthy of rapt attention on a standalone basis individually. On top of that, when you connect the dots and bring them together as a working partnership, you have to admire and anticipate big changes that will arise, especially as the two fields collaboratively reinvent data strategies all told.
This is entirely tangible and real-world, not merely something abstract or obtuse.
I will first do a quick overview of generative AI. If you are already versed in generative AI, perhaps do a fast skim on this portion.
Foundations Of Generative AI
Generative AI is the latest and hottest form of AI and has caught our collective devout attention for being seemingly fluent in undertaking online interactive dialoguing and producing essays that appear to be composed by the human hand. In brief, generative AI makes use of complex mathematical and computational pattern-matching that can mimic human compositions by having been data-trained on the text and other content found on the Internet. For my detailed elaboration on how this works see the link here.
In it, he explores how we can make better, scientifically informed predictions about the world around us, using maths. “Mathematics can provide us with the objective tools to bypass the foibles of our own biology – the limitations imposed by our own thought processes, the compulsions that ultimately make us human, but let us down when it comes to making inferences about the world around us,” he writes. “They are humanity’s shortcuts: the preconceptions and cognitive biases, refined over millennia of evolution, that all too often lead us astray when we try to apply our brain’s old rules to our society’s new environments.”
No matter how tempting it is to think, “Ooh, that’s a bit spooky” when faced with a completely random coincidence or chance occurrence, we should all be expecting unusual things to happen all the time, he says.
Yates describes a person who, when browsing in a secondhand bookshop far from where they grew up, opens a copy of their favourite children’s book, only to find their own name inscribed inside. Yet, he says, “the law of truly large numbers” dictates that, just as someone wins the lottery almost every week, with enough opportunities, such extraordinary coincidences are far more likely to happen than you might think. “There are so many different types of coincidences that make us say: ‘Well, that’s extraordinary.’ But it’s not unlikely that some of them happen to us every so often.”
With artificial intelligence poised to assist in profound scientific discoveries that will change the world, Cornell is leading a new $11.3 million center focused on human-AI collaboration that uses mathematics as a common language.
The Scientific Artificial Intelligence Center, or SciAI Center, is being launched with a grant from the Office of Naval Research and is led by Christopher J. Earls, professor of civil and environmental engineering at Cornell Engineering. Co-investigators include Nikolaos Bouklas, assistant professor of mechanical and aerospace engineering at Cornell Engineering; Anil Damle, assistant professor of computer science in the Cornell Ann S. Bowers College of Computing and Information Science; and Alex Townsend, associate professor of mathematics in the College of Arts and Sciences. All of the investigators are field faculty members of the Center for Applied Mathematics.
With the advance of AI systems – built with tangled webs of algorithms and trained on increasingly large sets of data – researchers fear AI’s inner workings will provide little insight into its uncanny ability to recognize patterns in data and make scientific predictions. Earls described it as a situation at odds with true scientific discovery.
A new study explored the causal role that music engagement has on student achievement in mathematics—and they found a significant benefit.
Researchers believe that music can make math more enjoyable, keep students engaged, and help ease their fear or anxiety about topics like fractions. The addition of music may even motivate kids to appreciate math and want to learn more.
A typical technique for integrating music into math lessons for young children involves clapping to songs with different rhythms learning numbers, and equating fractions to musical notes.
“Some men just want to watch the world burn.” Zachary Kallenborn discusses acts of existential terrorism, such as the Tokyo subway sarin attack by Aum Shinrikyo in 1995, which killed or injured over 1,000 people.
Zachary kallenborn is a policy fellow in the center for security policy studies at george mason university, research affiliate in unconventional weapons and technology at START, and senior risk management consultant at the ABS group.
Zachary has an MA in Nonproliferation and Terrorism Studies from Middlebury Institute of International Studies, and a BS in Mathematics and International Relations from the University of Puget Sound.
His work has been featured in numerous international media outlets including the New York Times, Slate, NPR, Forbes, New Scientist, WIRED, Foreign Policy, the BBC, and many others.
Forbes: New Report Warns Terrorists Could Cause Human Extinction With ‘Spoiler Attacks’
https://www.forbes.com/sites/davidhambling/2023/06/23/new-re…r-attacks/
Schar School Scholar Warns of Existential Threats to Humanity by Terrorists.