BrainAvatar brain mapping live electroencephalographic imaging and neurofeedback
Category: mapping – Page 3
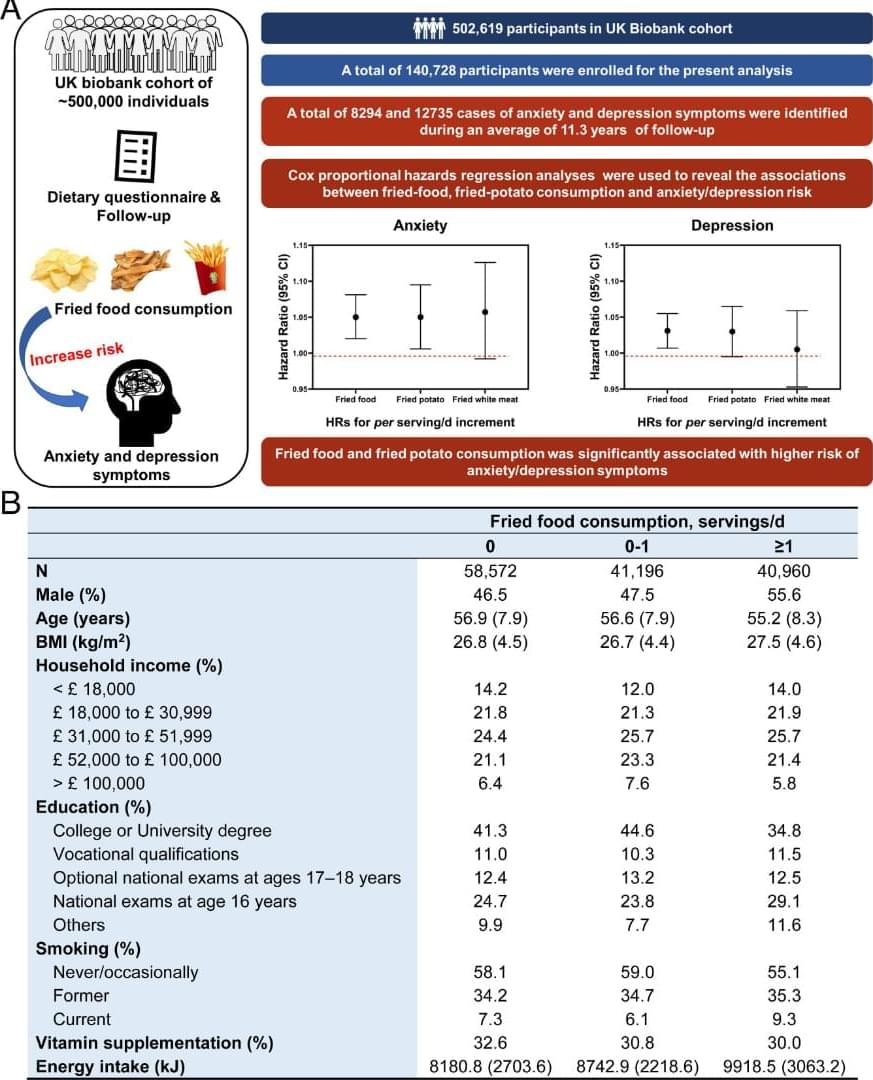
High fried food consumption impacts anxiety and depression due to lipid metabolism disturbance and neuroinflammation
When zebrafish are relocated to a new environment, they seek protection by diving and staying at the safety home, until they feel safe enough to explore the unfamiliar environment (26). The swimming trajectories showed that zebrafish in the control group can swiftly explore and adapt to the novel environment, but chronic exposure to acrylamide reduces the ability to adapt to the unfamiliar environment (Fig. 3 A). Visualized heatmaps showed significant changes in swimming trajectories of zebrafish in the acrylamide exposure groups compared with those in the control group (Fig. 3 B). Furthermore, we found the swimming time and distance ratios in Zone 1 exhibited a dose-dependent decreasing trend in acrylamide exposure groups. Chronic exposure to acrylamide (0.5 mM) significantly decreased both swimming time and distance in Zone 1 and increased those in Zone 2 (Fig. 3 C and D). We recorded the novel object exploration test to visualize the behavioral alteration between the control and each acrylamide-treated group (Movie S3). The movie displays that zebrafish in the control group could swiftly explore and adapt to the novel environment, but chronic exposure to acrylamide reduced the ability to adapt to the unfamiliar environment, which indicated that acrylamide induces anxiety-and depressive-like behaviors by reducing exploration ability of zebrafish.
Moreover, the social preference test was used to assess sociality of zebrafish. Since the zebrafish are a group preference species, they frequently swim by forming a school and swim closely to one another (27). In the current social preference test, representative radar maps and visualized heatmaps exhibited significant changes of preference in swimming trajectories of zebrafish in acrylamide exposure groups compared to those in the control group, indicating that chronic exposure to acrylamide remarkably impairs the sociality of zebrafish (Fig. 3 E–G). For detailed parameters of behavioral trajectories, chronic exposure to acrylamide (0.5 mM) significantly increased both swimming time and distance ratios in the left zone and decreased those in the right zone (Fig. 3 H and I). Notably, chronic exposure to acrylamide (0.5 mM) significantly elevated traversing times and number of crossing the middle line (Fig. 3 J and K).
You don’t need to speak—AI reads your face! | Privacy is no longer a right—it’s a myth
AI surveillance, AI surveillance systems, AI surveillance technology, AI camera systems, artificial intelligence privacy, AI tracking systems, AI in public surveillance, smart city surveillance, facial recognition technology, real time surveillance ai, AI crime prediction, predictive policing, emotion detecting ai, AI facial recognition, privacy in AI era, AI and data collection, AI spying tech, surveillance capitalism, government surveillance 2025, AI monitoring tools, AI tracking devices, AI and facial data, facial emotion detection, emotion recognition ai, mass surveillance 2025, AI in smart cities, china AI surveillance, skynet china, AI scanning technology, AI crowd monitoring, AI face scanning, AI emotion scanning, AI powered cameras, smart surveillance system, AI and censorship, privacy and ai, digital surveillance, AI surveillance dangers, AI surveillance ethics, machine learning surveillance, AI powered face id, surveillance tech 2025, AI vs privacy, AI in law enforcement, AI surveillance news, smart city facial recognition, AI and security, AI privacy breach, AI threat to privacy, AI prediction tech, AI identity tracking, AI eyes everywhere, future of surveillance, AI and human rights, smart cities AI control, AI facial databases, AI surveillance control, AI emotion mapping, AI video analytics, AI data surveillance, AI scanning behavior, AI and behavior prediction, invisible surveillance, AI total control, AI police systems, AI surveillance usa, AI surveillance real time, AI security monitoring, AI surveillance 2030, AI tracking systems 2025, AI identity recognition, AI bias in surveillance, AI surveillance market growth, AI spying software, AI privacy threat, AI recognition software, AI profiling tech, AI behavior analysis, AI brain decoding, AI surveillance drones, AI privacy invasion, AI video recognition, facial recognition in cities, AI control future, AI mass monitoring, AI ethics surveillance, AI and global surveillance, AI social monitoring, surveillance without humans, AI data watch, AI neural surveillance, AI surveillance facts, AI surveillance predictions, AI smart cameras, AI surveillance networks, AI law enforcement tech, AI surveillance software 2025, AI global tracking, AI surveillance net, AI and biometric tracking, AI emotion AI detection, AI surveillance and control, real AI surveillance systems, AI surveillance internet, AI identity control, AI ethical concerns, AI powered surveillance 2025, future surveillance systems, AI surveillance in cities, AI surveillance threat, AI surveillance everywhere, AI powered recognition, AI spy systems, AI control cities, AI privacy vs safety, AI powered monitoring, AI machine surveillance, AI surveillance grid, AI digital prisons, AI digital tracking, AI surveillance videos, AI and civilian monitoring, smart surveillance future, AI and civil liberties, AI city wide tracking, AI human scanner, AI tracking with cameras, AI recognition through movement, AI awareness systems, AI cameras everywhere, AI predictive surveillance, AI spy future, AI surveillance documentary, AI urban tracking, AI public tracking, AI silent surveillance, AI surveillance myths, AI surveillance dark side, AI watching you, AI never sleeps, AI surveillance truth, AI surveillance 2025 explained, AI surveillance 2025, future of surveillance technology, smart city surveillance, emotion detecting ai, predictive AI systems, real time facial recognition, AI and privacy concerns, machine learning surveillance, AI in public safety, neural surveillance systems, AI eye tracking, surveillance without consent, AI human behavior tracking, artificial intelligence privacy threat, AI surveillance vs human rights, automated facial ID, AI security systems 2025, AI crime prediction, smart cameras ai, predictive policing technology, urban surveillance systems, AI surveillance ethics, biometric surveillance systems, AI monitoring humans, advanced AI recognition, AI watchlist systems, AI face tagging, AI emotion scanning, deep learning surveillance, AI digital footprint, surveillance capitalism, AI powered spying, next gen surveillance, AI total control, AI social monitoring, AI facial mapping, AI mind reading tech, surveillance future cities, hidden surveillance networks, AI personal data harvesting, AI truth detection, AI voice recognition monitoring, digital surveillance reality, AI spy software, AI surveillance grid, AI CCTV analysis, smart surveillance networks, AI identity tracking, AI security prediction, mass data collection ai, AI video analytics, AI security evolution, artificial intelligence surveillance tools, AI behavioral detection, AI controlled city, AI surveillance news, AI surveillance system explained, AI visual tracking, smart surveillance 2030, AI invasion of privacy, facial detection ai, AI sees you always, AI surveillance rising, future of AI spying, next level surveillance, AI technology surveillance systems, ethical issues in AI surveillance, AI surveillance future risks.
Your Brain Maps Actions Like It Maps Space
A new study shows that the human brain organizes action-outcome associations in cognitive maps, much like how it maps physical space for navigation.
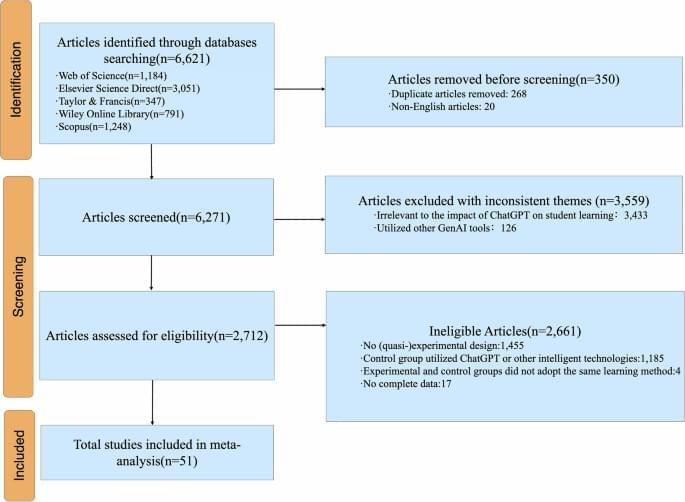
The effect of ChatGPT on students’ learning performance, learning perception, and higher-order thinking: insights from a meta-analysis
*An S, Zhang S, Guo T, Lu S, Zhang W, Cai Z (2025) Impacts of generative AI on student teachers’ task performance and collaborative knowledge construction process in mind mapping-based collaborative environment. Comput Educ 227. https://doi.org/10.1016/j.compedu.2024.105227.
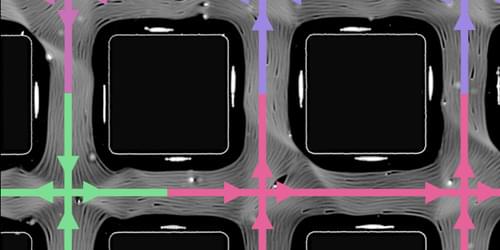
Active Fluids Solve Icy “Six-Vertex” Model
Researchers demonstrate an active-fluid system whose behaviors map directly to predictions of the six-vertex model—an exactly solvable model that was originally developed to explain the behavior of ice.
Active fluids—collections of self-propelled agents such as bacteria, cells, or colloids—consume energy to move, flowing without being pushed [1]. These materials break the conventional rules of fluid dynamics, as they can flow spontaneously, switch direction without apparent cause, and organize into complex patterns with no external control. Active fluids were initially studied to understand the collective dynamics observed in biological systems. Now they offer a rich playground for exploring nonequilibrium physics. Yet, in the ever-expanding universe of active-fluid physics, it is rare to find an experimental system that maps precisely onto a mathematically exact model.

Mapping memory: Protein tracking technique reveals synaptic changes during learning
A team of Harvard researchers have unveiled a way to map the molecular underpinnings of how learning and memories are formed, a new technique expected to offer insights that may pave the way for new treatments for neurological disorders such as dementia.
“This technique provides a lens into the synaptic architecture of memory, something previously unattainable in such detail,” said Adam Cohen, professor of chemistry and chemical biology and of physics and senior co-author of the research paper, published in Nature Neuroscience.
Memory resides within a dense network of billions of neurons within the brain. We rely on synaptic plasticity—the strengthening and modulation of connections between these neurons—to facilitate learning and memory.
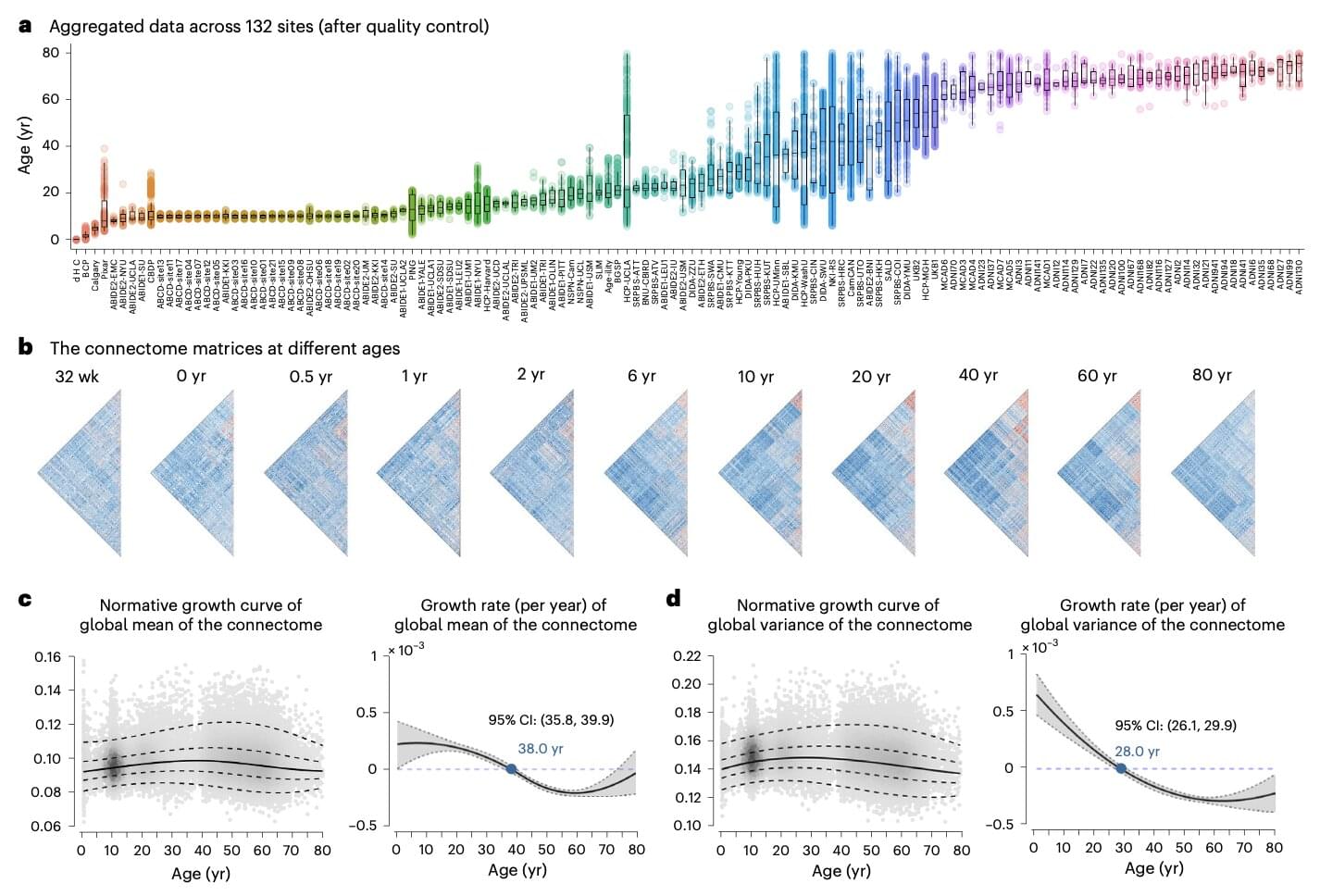
Large-scale study explores lifespan changes in the human brain’s functional connectivity
From birth to the last moments of life, the human brain is known to change and evolve significantly, both in terms of its physical organization (i.e., structural connectivity) and the coordination between different brain regions (i.e., functional connectivity). Mapping and understanding the brain’s evolution over time is of crucial importance, as it could also shed light on differences in the brains of individuals who develop various mental health disorders or experience an aging-related cognitive decline.
Researchers at Beijing Normal University and other institutes in China recently carried out a large-scale study to gather new insights into how the brain’s functional connectivity of humans worldwide changes over the course of their lifespan. Their paper, published in Nature Neuroscience, unveils patterns in the evolution of the brain that could inform future research focusing on a wide range of neuropsychiatric and cognitive disorders.
“Functional connectivity of the human brain changes through life,” wrote Lianglong Sun, Tengda Zhao and their colleagues in their paper. “We assemble task-free functional and structural magnetic resonance imaging data from 33,250 individuals at 32 weeks of postmenstrual age to 80 years from 132 global sites.”
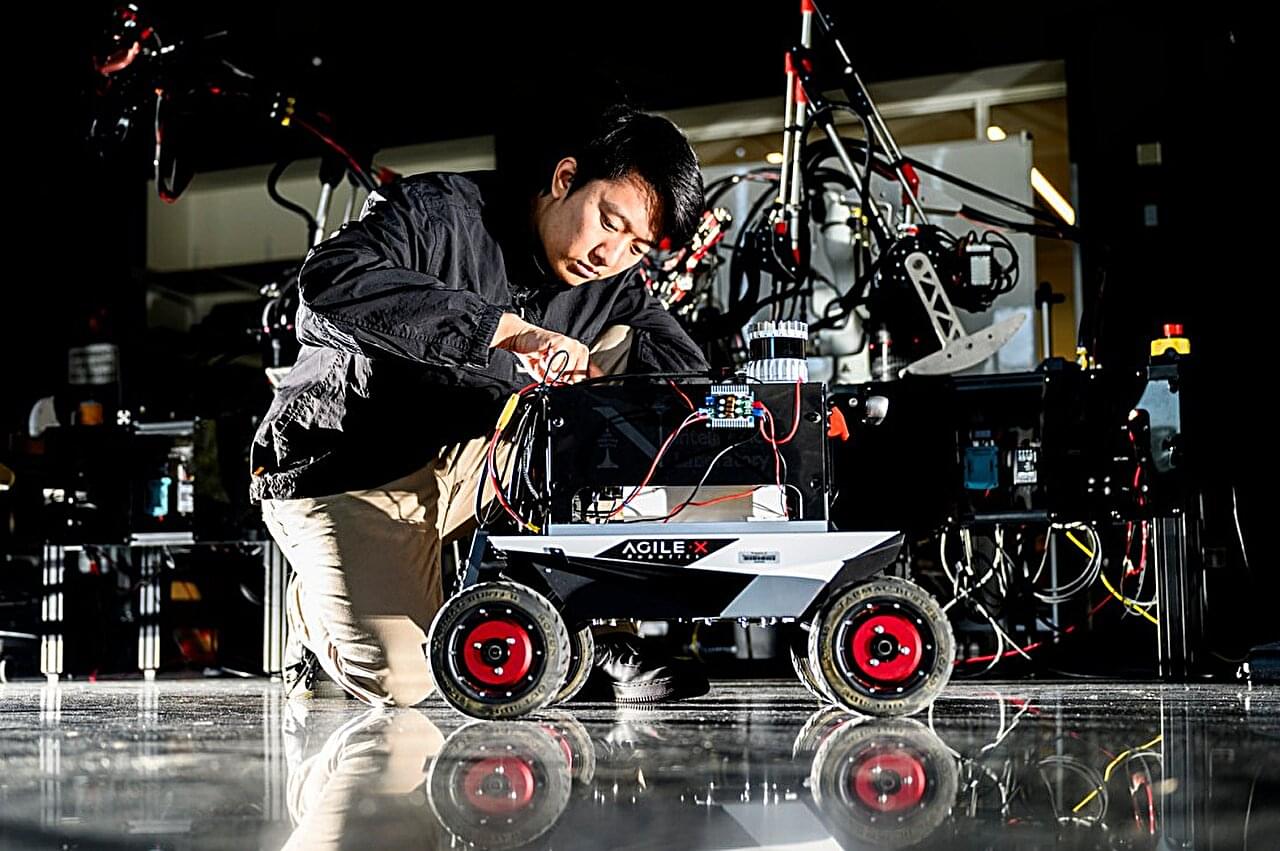
Robotics researchers develop algorithms that make mobile navigation more efficient
Delivery robots made by companies such as Starship Technologies and Kiwibot autonomously make their way along city streets and through neighborhoods.
Under the hood, these robots—like most mobile robots in use today—use a variety of different sensors and software-based algorithms to navigate in these environments.
Lidar sensors—which send out pulses of light to help calculate the distances of objects—have become a mainstay, enabling these robots to conduct simultaneous localization and mapping, otherwise known as SLAM.

Parallel activity in orbitofrontal cortex and hippocampus shapes cognitive maps and schemas, study suggests
As humans and other animals navigate their surroundings and experience different things, their brain creates so-called cognitive maps, which are internal representations of environments or tasks. These mental maps are eventually generalized into schemas, frameworks that organize information acquired through experience and can later guide decision-making.
Various past neuroscience and psychology studies have tried to better understand the neural processes and brain regions that support the formation of these internal representations. Insight into these mechanisms could, in turn, shed light on the underpinnings of learning and decision-making.
Two brain regions that have been found to play a role in forming internal representations of experiences are the orbitofrontal cortex (OFC) and the hippocampus (HC). Among other functions, the OFC supports reward-based learning and decision-making. At the same time, the HC contributes to spatial navigation and the formation and retrieval of memories.