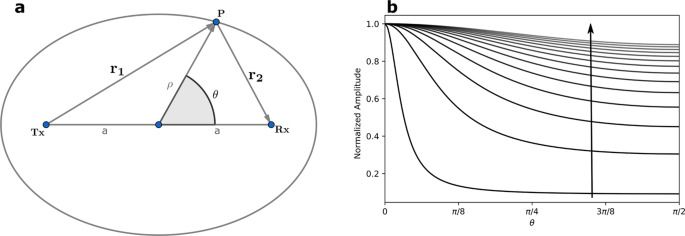
Advanced uses of time in image rendering and reconstruction have been the focus of much scientific research in recent years. The motivation comes from the equivalence between space and time given by the finite speed of light c. This equivalence leads to correlations between the time evolution of electromagnetic fields at different points in space. Applications exploiting such correlations, known as time-of-flight (ToF)1 and light-in-flight (LiF)2 cameras, operate at various regimes from radio3,4 to optical5 frequencies. Time-of-flight imaging focuses on reconstructing a scene by measuring delayed stimulus responses via continuous wave, impulses or pseudo-random binary sequence (PRBS) codes1. Light-in-flight imaging, also known as transient imaging6, explores light transport and detection2,7. The combination of ToF and LiF has recently yielded higher accuracy and detail to the reconstruction process, especially in non-line-of-sight images with the inclusion of higher-order scattering and physical processes such as Rayleigh–Sommerfeld diffraction8 in the modeling. However, these methods require experimental characterization of the scene followed by large computational overheads that produce images at low frame rates in the optical regime. In the radio-frequency (RF) regime, 3D images at frame rates of 30 Hz have been produced with an array of 256 wide-band transceivers3. Microwave imaging has the additional capability of sensing through optically opaque media such as walls. Nonetheless, synthetic aperture radar reconstruction algorithms such as the one proposed in ref. 3 required each transceiver in the array to operate individually thus leaving room for improvements in image frame rates from continuous transmit-receive captures. Constructions using beamforming have similar challenges9 where a narrow focused beam scans a scene using an array of antennas and frequency modulated continuous wave (FMCW) techniques.
In this article, we develop an inverse light transport model10 for microwave signals. The model uses a spatiotemporal mask generated by multiple sources, each emitting different PRBS codes, and a single detector, all operating in continuous synchronous transmit-receive mode. This model allows image reconstructions with capture times of the order of microseconds and no prior scene knowledge. For first-order reflections, the algorithm reduces to a single dot product between the reconstruction matrix and captured signal, and can be executed in a few milliseconds. We demonstrate this algorithm through simulations and measurements performed using realistic scenes in a laboratory setting. We then use the second-order terms of the light transport model to reconstruct scene details not captured by the first-order terms.
We start by estimating the information capacity of the scene and develop the light transport equation for the transient imaging model with arguments borrowed from basic information and electromagnetic field theory. Next, we describe the image reconstruction algorithm as a series of approximations corresponding to multiple scatterings of the spatiotemporal illumination matrix. Specifically, we show that in the first-order approximation, the value of each pixel is the dot product between the captured time series and a unique time signature generated by the spatiotemporal electromagnetic field mask. Next, we show how the second-order approximation generates hidden features not accessible in the first-order image. Finally, we apply the reconstruction algorithm to simulated and experimental data and discuss the performance, strengths, and limitations of this technique.