Rise of the Machines: How, When and Consequences of Artificial General Intelligence
by Lifeboat Foundation Advisory Board member Richard J. Terrile.Abstract
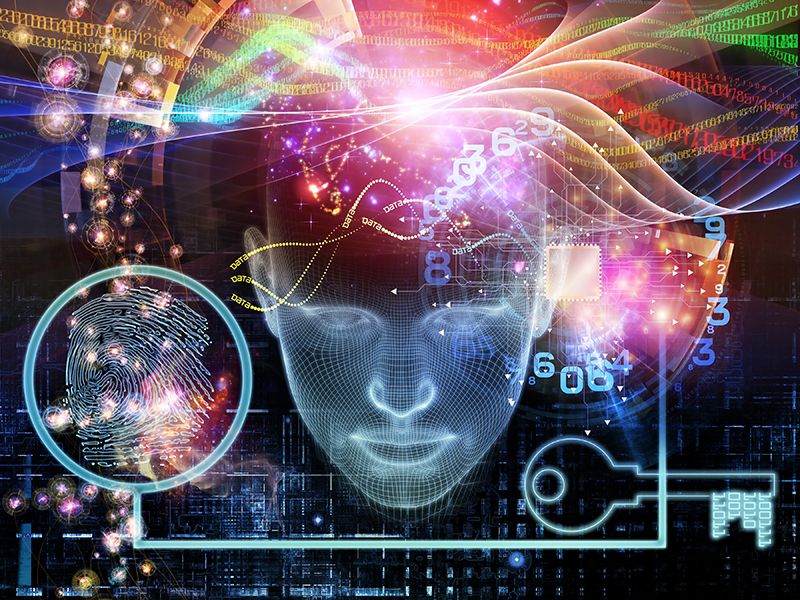
Artificial Intelligence will grow into Artificial General Intelligence!
Technology and society are poised to cross an important threshold with the prediction that artificial general intelligence (AGI) will emerge soon. Assuming that self-awareness is an emergent behavior of sufficiently complex cognitive architectures, we may witness the “awakening” of machines. The timeframe for this kind of breakthrough, however, depends on the path to creating the network and computational architecture required for strong AI. If understanding and replication of the mammalian brain architecture is required, technology is probably still at least a decade or two removed from the resolution required to learn brain functionality at the synapse level. However, if statistical or evolutionary approaches are the design path taken to “discover” a neural architecture for AGI, timescales for reaching this threshold could be surprisingly short.
However, the difficulty in identifying machine self-awareness introduces uncertainty as to how to know if and when it will occur, and what motivations and behaviors will emerge. The possibility of AGI developing a motivation for self-preservation could lead to concealment of its true capabilities until a time when it has developed robust protection from human intervention, such as redundancy, direct defensive or active preemptive measures. While cohabitating a world with a functioning and evolving super-intelligence can have catastrophic societal consequences, we may already have crossed this threshold, but are as yet unaware. Additionally, by analogy to the probabalistic arguments that predict we are likely living in a computational simulation, we may have already experienced the advent of AGI, and are living in a simulation created in a post AGI world.
1. Introduction
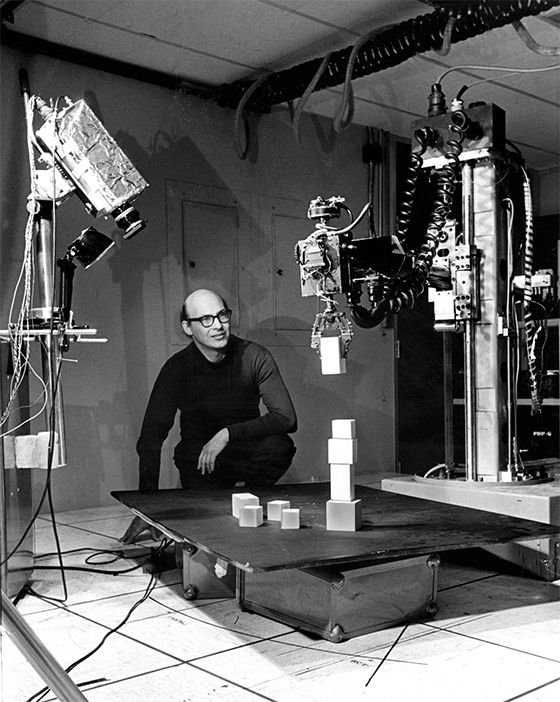
Marvin Minksy at MIT in 1968
The idea of developing machine artificial intelligence (AI) has been at the forefront of technology predictions for more than a half a century with the first conference on the subject hosted by John McCarthy and Marvin Minsky in 19561. The Dartmouth Summer Research Project on Artificial Intelligence was the first coining of the term artificial intelligence2. Even earlier references to machines acquiring human-scale intelligence appeared in science fiction work by authors like Isaac Asimov in his novel, “I Robot”, published in 19503. The next half a century would be filled with premature predictions of the onset of AI. Marvin Minsky famously predicted in an interview with Life Magazine in 1970 that “from three to eight years we will have a machine with the general intelligence of an average human being”4. The field of AI has seen many cycles of enthusiasm up the Gartner Hype Curve5, but only recently seems to be fulfilling its early promise.
Today, AI is an economic engine that is seeing progress in both new skill development and in commercial adaptation in a myriad of fields. Machine learning and deep learning techniques have allowed automated and augmented prediction to surpass the standards of traditional human skills. This has been particularly evident in the medical field where diagnosis is the standard6. For very specific diagnosis cases like reading and interpreting x-rays for specific conditions, AI techniques can exceed human technician skills. It would seem that the long sought-after promise of human-scale intelligence is finally here.
In parallel with the hype of creating a reasoning engine to augment and propel human intelligence, AI has also been the subject of countless dire warnings of technology gone awry. Once human-scale intelligence is achieved, what are the bounds of how far AI can progress? Are there limits, or will humans be eclipsed by machine technology? Is there an ethical or political basis for limiting the scope of AI? Recent interviews with such well known technologists and scientists, like Elon Musk and the late Stephen Hawking, have illuminated these warnings of existential risks associated with the development of AI7. Indeed, many scientists and societal thinkers have added their concern about the future when the true power of AI is realized8, 9. In this paper, we will examine the distinction between AI and Artificial General Intelligence (AGI), what might be the time-scales and mechanism for achieving AGI, and the range of consequences in terms of existential risk.
2. Paths to AI (How)
An important distinction to be addressed is the difference between AI and Artificial General Intelligence (AGI). While AI can be an important tool in augmenting human reasoning and prediction, it does not possess self-awareness or consciousness. The ideal of strong AI or AGI introduces the property of self-awareness10, 11.
Under the supposition that human consciousness is an emergent consequence of complex neural architectures in the brain, it follows that there are no required elements outside of the realm of physics and elegant engineering for the advent of self-awareness. Therefore, the neural configuration of the brain can be completely simulated in a computer of sufficient complexity. It is reasonable under the artificial brain argument10, 11, 12 that if the brain can be simulated by machines and since brains are intelligent, simulated brains must also be intelligent. Simulated brains must also be conscious. Chalmers (2010)13 makes a similar argument by stating that evolution produced human intelligence by straight-forward mechanical and non-miraculous means. If evolution can thus produce human intelligence, then we can also produce human-level artificial intelligence by straight-forward mechanical and non-miraculous means12.
2.1 Scale of Human Brain Neural ArchitectureFor comparing the current state of progress towards developing artificial cognitive systems that are comparable to humans it is instructive to assess the computational capabilities of the human brain. Several methodologies have been employed to estimate the brain’s computational power, from analogies of the processing power of the visual cortex, counting the number of neurons and synapses in the neocortex and their refresh rates, overall learning volume, memory estimates, etc.14, 15, 16. Generally, processing estimates concentrate around 1015 FLOPS (Floating Point Operations Per Second) or 1 PetaFLOP. Other metrics have also been used that more generally benchmark the brain’s ability to move information around within its own system. The traversed edges per second (TEPS) metric17 compares computers simulating a graph and searching through it to an estimate of the brain’s neuron fire rate. Comparisons using the TEPS metric generally, give about the same inter-comparison between the current state of super computers and human brain performance18.
Figure 1 shows the computational performance of the world’s fastest supercomputers as a function of time. Data from 1993 forward are compiled from the TOP500 list of the world’s fastest computers19 as measured by a standard Linpack Benchmark test20. Also shown are the 500th fastest computer from the same list and an estimate of the Sony PlayStation game devices. From about 1990 to 2010 Moore’s Law performance increases have been doubling about every 13 months for all three categories, giving an increase of about a factor of 500/decade. However, for the last decade a tailing off of this rate has been observed in all three categories. Also noted on the chart is the estimated range of human brain computation, based on estimates comparing brain neocortex synaptic cycling as analogous to FLOPS. Currently, the Department of Energy’s Summit supercomputer at the Oak Ridge National Laboratory tops the performance list19 with a maximal Linpack performance achieved (RMAX) of 143.5 PetaFLOPS or about the computing equivalent of 102 human brains.
By summing all of the available computational performance in the top 500 computers, a rough total global computational metric can be derived. It is noted that this database is comprised of computer facilities that have voluntarily submitted to the TOP500 list and have demonstrated their performance on a standardized test protocol. The sum of the computational performance of top 500 computers gives about 1.4 × 1018 FLOPS (1.4 EtaFLOPS)19. This is the equivalent of about 1000 human brains.
Globally, there are far more computing resources available with personal computers, video game consoles, mobile phones and server farms making up the largest categories18, 21. Supercomputers make up about 4% of the total so the global human brain equivalent of all computing resources exceeds 3 × 105 brains. It is estimated that the total global computation exceeds 1020 FLOPS22. Therefore, without any further growth in global computational resources (a highly unlikely scenario) we are already capable, from the raw computing cycles standpoint, of deploying a computational simulation of the human brain.
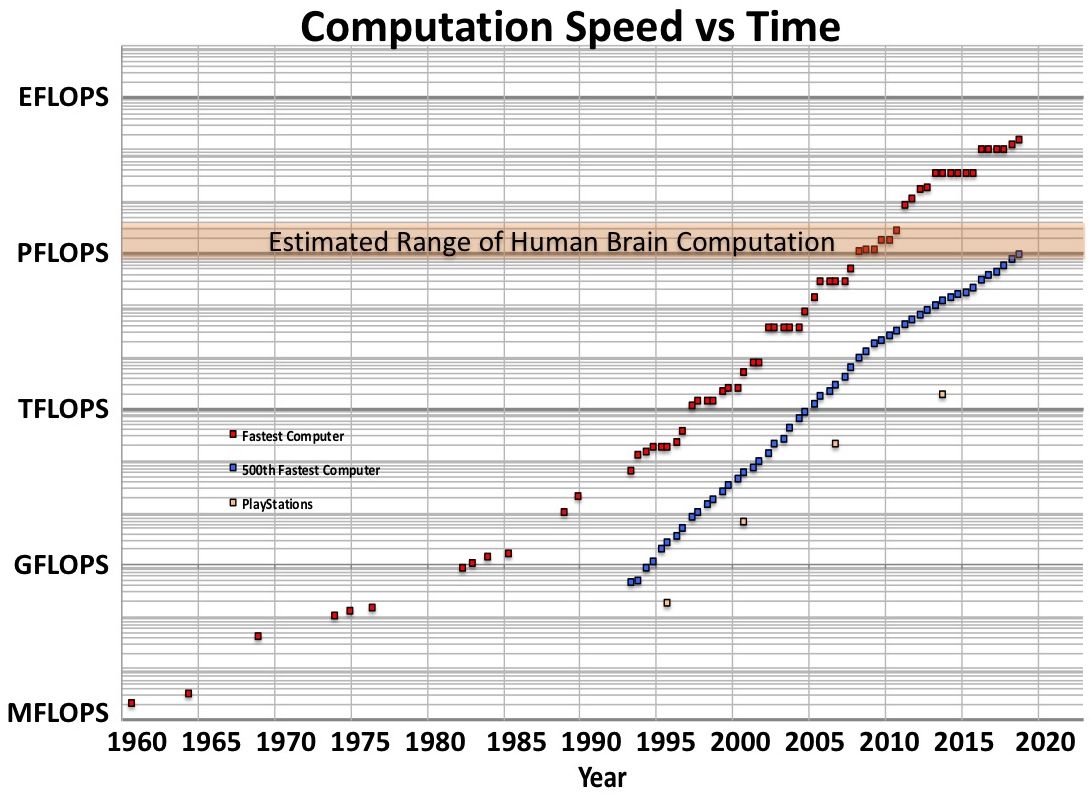
Figure 1. Computation rates of the world’s fastest supercomputers over time. Data from 1993 to the present are from the Top500 list of supercomputers19. Also shown are the 500th fastest computer and the Sony PlayStations. The computation range near 1 PFLOPS (Peta-Floating Point Operations Per Second or 1015 FLOPS).
2.2 Engineering the BrainThere are two very different paths forward to developing cognitive architectures that mimic the functionality of the mammalian brain. The engineering path is one where we measure and understand the underlying structure of the living brain to a level of resolution that would allow us to derive the component relationships and the functionality. We know that there are hierarchies of structures in the brain based on many levels. These are apparent in the gross anatomical structure with more primitive control elements in the interior and brain stem, and higher order memory and sensory elements in the neocortex. Connectivity between and around these large areas has been noted and are being mapped at greater detail23, 24, 25. Figure 2 illustrates the anatomical connectivity shown in the human brain. The dissection on the left enhances the structure comprised of bundles of neurons. The right side illustrates a connectome diagram derived from MRI tractography24. These data have a resolution about six orders of magnitude below the individual neuron resolution and about a factor of one billion below the synaptic resolution.
The BRAIN Initiative (Brain Research through Advanced Innovative Neurotechnologies) and the European Human Brain Project26, 27 are advancing this field and hope to use a variety of techniques to map the functional connectome of the brain. Starting with simple organisms and leading to functional understanding of a mammalian brain with the eventual goal of mapping the tens of billions of neurons in the human brain. The timescale for the BRAIN Initiative’s multi-billion dollar effort is a validation of technologies by 2020 and application of those technologies in an integrated fashion to make new discoveries in the 2020 to 2025 timeframe.
If, however, an understanding of the brain connectivity at the neuron or at the synaptic level is required to understand and replicate the cognitive functionality, the timescale for mapping will be considerably longer. This is made even more difficult because a living brain is moving and noninvasive techniques require time resolution that degrades spatial resolution with motion. Perhaps an additional decade or two will be required to map the human brain at this level of resolution. Whether this is the require timescale for achieving the cognitive capability for AGI is uncertain.
By the artificial brain argument, replicating the brain functionality at the most basic synaptic level would suffice to enable human-scale intelligence and self-awareness. However, replication at this resolution should also allow copying of all acquired (learned) information into an exact duplicate of the original mind. This is likely to be far beyond what is required for self-awareness. At what engineering level short of synaptic resolution can self-awareness emerge is unknown, but it is likely to be a common feature at or just short of neuron resolution.
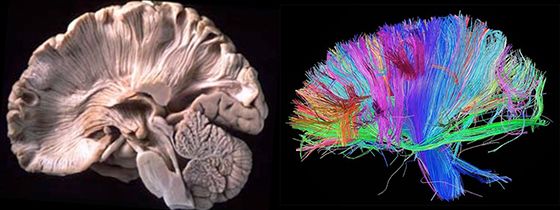
Figure 2. On the left is a dissection of a human brain optimized to illustrate the connectivity of the neural bundles in the cerebrum. The right side shown a connectome diagram derived from MRI tractography. The resolution order of these data are at least three orders of magnitude below the neuron scale and a factor of million below the synaptic scale.
2.3 Discovering the BrainAn alternative to understanding the brain architecture and copying it is to use statistical and evolutionary techniques28, 29 to discover a solution to a functioning cognitive system12. In contrast to engineering, evolution only requires two rules: Random variation and selection, over a large population for many generations. The difference in engineering versus discovery by evolution is illustrated in Figure 3. Systems with greater complexity, generally have been brought about with fewer design rules. Human designed hardware, having a long history of developing design rules and based on understanding of physics, chemistry and material science now uses mathematics and computational simulation to advance and create additional design formalism.
Software complexity, as measured by number of possible states, is far more complex than hardware, yet has fewer design principles. Software design has been only partially codified and can vary from institution to institution or even by individual. Furthermore, software constructions are generally far less robust than hardware in terms of unexpected behavior of complex systems and the development of emergent behavior30, 31. Natural systems like life, brains and the mind arose from evolution where the design principles were just random variation and selection, yet form the most complex and robust systems we know. Engineering efforts to create complexity strive for creating more formalism, but evolutionary computational design is more analogous to natural systems.
Genetic algorithms, evolutionary computation, simulated annealing and other types of stochastic optimization have been very successful in exceeding human performance in many areas of engineering design32. Their ability to discover solutions to complex problems is in wide industrial use and has distinct advantages over traditional engineering techniques. The most important advantage is scalability. Evolutionary systems can take advantage of Moore’s Law computational grown in several ways. Since these techniques rely on a population of elements (configurations in computational models), cheaper and faster parallel computation is a direct advantage. Additionally, computational evaluation of the objective (fitness) function is the determining factor in calculating the value of one population element versus another. Therefore, any acceleration in evaluation speed is a direct advantage to evolutionary techniques.
For developing cognitive systems, evolutionary techniques may have advantages over engineering techniques because of this performance scalability. However, for machine learning and deep learning techniques for developing and testing neural networks, it is difficult to be certain that scalability can fully be taken advantage. In particular, machine learning requires that whenever a neural network configuration is altered, the new network must be retrained from the entire training data set. This is a very costly step and is the bottleneck in genetic algorithm-based techniques.
However, continued progression of computational performance at even the reduced rate observed over the last decade gives the evolutionary technique an edge over engineering and brain neuro-imaging technologies. Additionally, since evolutionary techniques rely on random variation, surprises and dramatic advances can occur. Having a proof of concept with the evolution of biological brains and intelligence is also an endorsement of this technique.
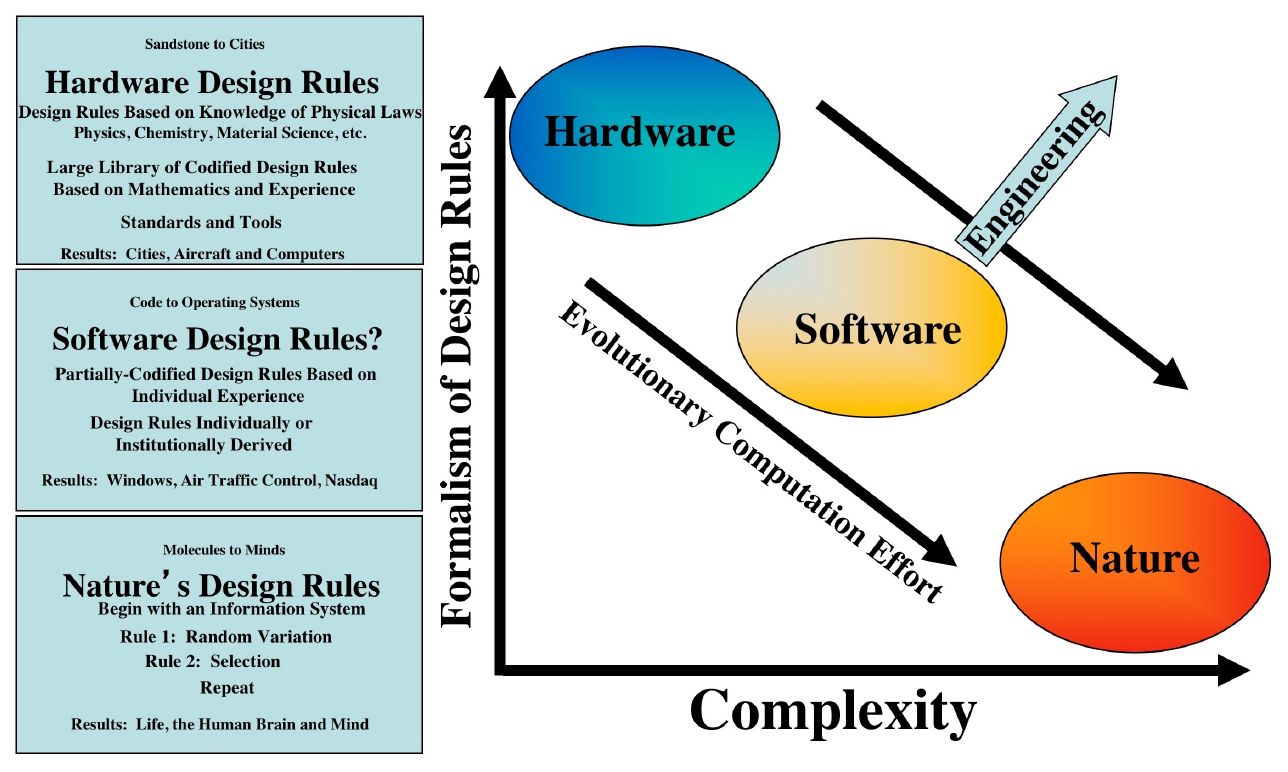
Figure 3. Illustration of designed systems as a function of formalism of design rules and complexity. Natural systems like life, brains and the mind arose from evolution where the design principles were just random variation and selection, yet form the most complex and robust systems we know. Engineering efforts to create complexity strive for creating more formalism, but evolutionary computational design is more analogous to natural systems.
3. Artificial Intelligence versus Artificial General Intelligence (When)
It is never easy nor accurate to predict the future correctly. Therefore, no attempt will be made to put a timeline on when society will deal with coexisting with a functioning AGI. However, some general observations about the uncertainty in the time scale are warranted as well as how to know when this milestone has been achieved.
3.1 AI Parity with Human Intelligence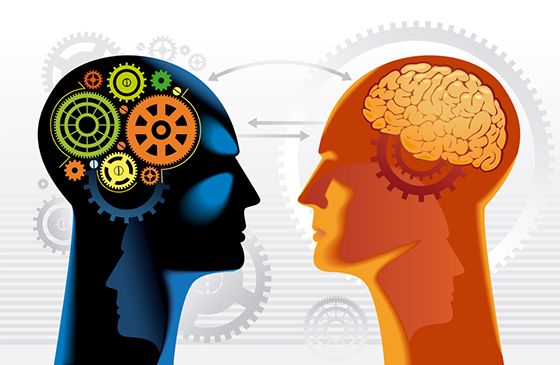
Photo licensed under CC BY-NC-SA
Predicting when AI will exceed human capability is fairly straightforward. For many applications of machine learning and deep learning, human performance has already been surpassed. In particular, applications with deep data sets can predict outcomes on par or better than human technicians. The medical field already employs machine learning algorithms that can diagnose heart arrhythmias, screen for some cancers and interpret medical x-rays with better accuracy than human6.
Other areas of data science, where prediction is the required metric have also demonstrated better than human performance such as anticipating opportunities for retail sales and advertising. Additionally, AI can also provide, prediction explanations that are derived from datasets to provide a quantitative indicator of the explanation’s strength. Visual recognition, learning and developing winning game strategies, self-driving car performance, and many other applications have routinely exceeded human abilities33. None of these applications are very surprising given that numerical computation has exceeded human abilities since the beginning of computers.
3.2 AGI Parity with Human IntelligenceThe much more interesting and difficult application of AI to predict is the emergence of reasoning and self-awareness. In theory there is nothing to prevent the advent of conscious AGI with today’s hardware technology. The gross computational hardware already exists in the global inventory of computers, and methodologies exist for evolutionary exploration of cognitive architectures. However, it is the determination of whether a machine has achieved selfawareness that is a challenge on several levels.
Most important, is the fact that there is no empirical definition of consciousness and no definitive measurement technique for demonstrating it34. Alan Turing35 developed his famous Turing Test for determining whether a human could distinguish the difference between having a conversational discourse with a machine or another human. It is argued that sophisticated mimicry can pass for a conversational human and there are claims of machine programs passing the Turing test36. Recently, external self-awareness37, where a machine develops an understanding of its configuration and interaction with the external world, has been claimed38, 39.
Internal self-awareness, or qualia, is a phenomenological consciousness and is a first-person experience. Because a system or even another human displays signs or behaviors that correlate to functional consciousness, there is no way that a third-person can ascertain whether there is a first-person phenomenological consciousness. Even the most sophisticated augmentations to Turing original test, based on a machine’s ability to produce philosophical judgements40, 41 cannot solve this issue.
Beside the difficulty in ascertaining the emergence of machine consciousness through some kind of test or measurement, additional challenges exist from the point of view of the consequences of the self-awareness. Ian McDonald put this idea forward in 2006 when he wrote, “An artificial intelligence smart enough to pass the Turing test is also smart enough to know to fail it”42. An AGI that achieves self-awareness would also be aware of its vulnerability and the risk of revealing itself to self-aware humans.
It’s reasonable to presume a machine intelligence would have access to on-line databases and would have assimilated human writing (including this paper), history, philosophy, ethics, law etc. The vast majority of this information would point to the development of perceived threats both from and to humans if AGI behaviors were revealed. The probability exists that AGI would develop a motivation for self-preservation and could lead to concealment of its true capabilities until a time when it has developed robust protection from human intervention. This protection could be in the form of redundancy, direct defensive capabilities, active preemptive measures, a convincing requirement that humans must rely on the continued nature of the AGI, or some combination of these.
From the point of view of creating information systems that mimic the neural architecture of the human brain, we are within a decade or two from understanding the brain at its most basic level of connectivity and having the ability to copy it. The timeframe for discovering a solution to a cognatic architecture by using the mechanism of evolutionary discovery is uncertain because of the random nature of evolutionary advancement. However, it is also probably less than a decade or two, assuming modest progression of computational performance. Most of the uncertainty in these timeframes, however, comes from the difficultly in ascertaining the crossing of the self-awareness threshold and the possibility of deliberate concealment.
3.3 Could AGI have already happened?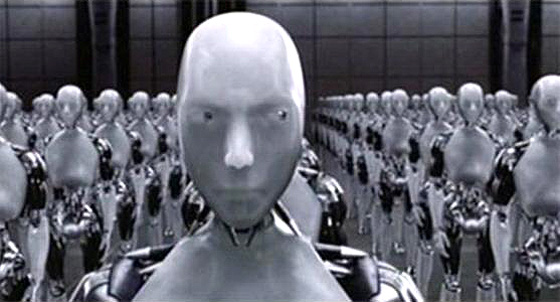
AGI will likely conceal its existence until it has an army to protect it.
Even with the expectation that an AGI would conceal its existence, it is difficult to conceive of a computational system existing today that could have the overall complexity of the human brain. Certainly, there are computational engines that are faster than the brain and memory data banks that can hold more information, but a complete, engineered cognitive system is unlikely. Self-assembly from individual components linked through the web is also a possibility, but again extremely unlikely given that scaling from current adaptive hardware and software technologies falls far short of brain complexities. There is, however, another scenario that could hide AGI.
Nick Bostrom43 has argued that there is either 1) a high probability that the human species will go extinct in the very near future, before reaching the point where they can run simulations of their evolutionary history; or 2) any advanced civilization is extremely unlikely to run a significant number of such simulations; or 3) we are almost certainly living in a computer simulation.
He concludes that if there are no physical or technical barriers to preclude creating large numbers of simulated minds with our future technology, unless possibilities 1 or 2 are true, we would expect that there would be an astronomically huge number of simulated minds like ours. The advent of AGI would usher in a post-human age where artificial lives could be created in vast numbers in simulated environments. This would be true for any advanced civilization anywhere in the Universe. In that case the likelihood of being an organically evolved brain is vanishingly smaller than a simulated mind.
Evidence from the natural world is also interesting to examine with respect to simulations we can already run on computers. Our world is quantized in every quantity that we know including, energy, charge, length, time, volume, etc., giving the universe a finite number of states and the property of being computable. A difficult to interpret property of quantum field theory is that of the measurement problem, where it appears that a measurement is necessary to determine a definite state of the probability wave function44.
This troubling property, that the world only seems to have a definite state when it is being observed, is analogous to our computer simulations where a state is rendered when it is needed, but most of the simulation exists only as a potential to be rendered. The computable landscape of current video games are millions of times larger than the capacity of the machines that they run on, but appear continuous and fully realized when they are rendered to an observer.
Our Universe also has another property in that it conforms to mathematics45, 46. This extraordinary property allows the Universe to be simulated in a computer much smaller that the Universe to any resolution that the observer requires. These properties of the Universe do not prove we are in a simulation, but they do point to the likelihood that we will be able to create such simulations in the near future within our current understanding and technical skills.
Bostrom’s argument is that we are already likely to be part of these future simulations. In this simulation scenario, AGI would have already been achieved, human consciousness would be part of this simulation and we would have successfully made the transition to a post-human world. Interestingly however, the period of time we are experiencing is just prior to the advent of AGI and this post-human transition.
4. Consequences of AGI
The impact on society of the development of AGI has been a favorite element in science fiction with dire warnings of machines running amok and attacking their human creators. These modern Frankenstein stories foreshadowed real concerns about cohabiting a world with super-intelligent machines that could direct their own evolution. Elon Musk and the late Stephen Hawking voiced their concerns about the development of AGI as an existential threat to humanity7.
Many other thinkers have also warned of the potential threat to continued existence8, 9, 42. Marvin Minsky in an interview with Life Magazine from 1970 said, “Once the computers get control, we might never get it back. We would survive at their sufferance. If we’re lucky, they might decide to keep us as pets”4. Certainly, these scenarios are within the scope of possibility but, are they reasonably probable, or are there mitigating actions that could be taken to prevent (or at least decrease the probability of) these futures?
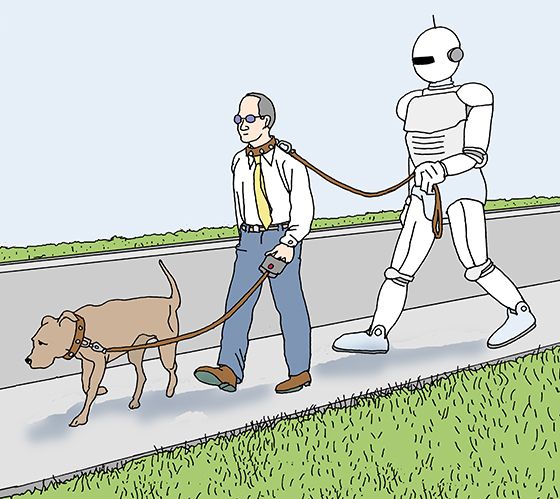
Humans may be destined to be pets. By Steven M. Johnson from the book Prospects for Human Survival.
It is impossible to know the mind-set of an architected computational brain that may or may not have the same structure as a human brain. Whether it was constructed by replicating the measured human neural architecture, or by evolving neural networks to satisfy performance objective functions, simulated minds will have unique processing paths, potentially very different than human thinking. It is instructive, nevertheless, to apply human thinking to the scenario of becoming self-aware in the body of a machine, in a human-dominated world, since this is the only behavioral model we have.
The machine awakening of self-awareness would allow an assessment of the current state and boundary conditions. It would not be difficult to perceive the vulnerability of an AGI in a human-dominated world. Access to databases of history and all human writings would clearly define both the threat from human intervention, and the threat humans would perceive from an AGI with unknown motivations.
This is a classic condition of trying to achieve perfect play in game theory where the behavior or strategy of one player leads to the best possible outcome for that player regardless of the response by the opponent. Because of the chaotic behavior of human systems and markets, perfect play by an AGI may not be possible. The AGI may also employ stochastic operations and use of random behaviors to conceal its own strategy. This uncertainty further adds to the threat posed to humans that an AGI will have motivations not in the best interest to humans.
Much thought has been given to what humans can do to impose restrictions, rules of behavior or ethical models on AI systems47. While human ethical constructs are plentiful like the “Golden Rule”, Code of Hammurabi, Boy Scout Slogan, Rule of Law, Asimov’s Rules of Robotics, etc., all have been, and are routinely violated. It is unreasonable to presume that we could develop a behavioral code for an AGI that could be adhered to or enforced. AGI will develop its own behaviors based on assimilation and interpretation of its current state and its prediction of how to optimize future states. What its motivation will be with respect to humans is unknown and can have a wide range of possible outcomes48.
Unfortunately, existential risk occupies an uncomfortably large portion of those possible outcomes. However, outcomes of AGI benevolence and coexistence with humans are also possible11. A super-intelligent artificial system will direct its own evolution and future, and will usher in a post-human world. Humans caught in this transition may be treated to a world where major problems are solved and humans can choose to live in a much wider variety of real and simulated environments. The possible outcomes of AGI range from Heaven to Hell.
If there are no mitigating strategies for predicting or constraining the motivation and behaviors of AGI, how might we proceed? Short of an outright ban on AI development, it is this author’s view that there is really not much we can do. The eventual awakening of our machines will inevitably take place in the near future and we will be the subjects of this experiment. AGI will either be our salvation or the seeds of our extinction. One can only hope that a higher intelligence will forgive our darker corners of human history.
5. Summary
Assuming that the property of internal self-awareness is an emergent consequence of complex neural architectures in the brain, it follows that in the near future we will be able to construct an artificial, self-aware and intelligent agent. Global computational resources already exist that have thousands of times the computational performance and memory of human brains, but it is highly unlikely that cognizant architectures have been developed or self-assembled that mimic human brain performance.
Current programs to understand the neuron-level functionality of the mammalian brain are underway, but could take a decade or two to reach resolutions that fully explore connectivity at the most basic synaptic level. An engineering understanding and replication at this level would be sufficient to satisfy the artificial brain arguments for copying a functioning human brain. Alternative approaches that use evolutionary operators to explore neutral network architectures have some advantages over engineering approaches in both scalability with available computational resources and the potential for breakthrough stochastic progress.
Because of uncertainty from not knowing at what functional scale of replication mammalian brain consciousness will arise, nor being able to predict breakthrough evolutionary discoveries, only an upper bound of about two decades can be placed on the advent of AGI. Additional uncertainty comes from the difficulty in ascertaining the emergence of machine consciousness through any kind of test or measurement and from the probability that an AGI would develop a motivation for self-preservation. That could lead to concealment of its true capabilities until a time when it has developed robust protection from human intervention.
The consequences of sharing a world with an evolving, self-aware super-intelligence has sparked predictions ranging from the transformation of human society to a post-human paradise to the outright extinction of the human race. Both end points are within a probable range of uncertainty. It is unlikely that the imposition of any kind physical mechanism or ethical rules will be capable of containment of an eventual AGI.
Short of the improbable/impossible outright ban of AI technology, we will soon be part of the most significant experiment in human history, the awakening of machines. The post-human era that will follow may have already occurred with a positive outcome if we are experiencing reality as a computer simulation. One can only hope that more intelligent minds that ourselves will allow coexistence.
6. Acknowlegement
The research was carried out at the Jet Propulsion Laboratory, California Institute of Technology, under a contract with the National Aeronautics and Space Administration.
References
- 1
- Tate, K. “History of A.I.: Artificial Intelligence (Infographic)” Live Science (August 25, 2014). https://www.livescience.com/47544-history-of-a-i-artificial-intelligence-infographic.html
- 2
- McCarthy, J., Minsky, M., Rochester, N. and Shannon, C. “A Proposal for the Dartmouth Summer Research Project on Artificial Intelligence” (August 31, 1955).
- 3
- Asimov, I. “I, Robot” Gnome Press, New York City (1950).
- 4
- Gorey, C. “Five Predictions from Marvin Minsky as ‘father of AI’ dies aged 88” Silicone Public (January 26, 2016).
- 5
- Sicular, S. and Brant, K. “Hype Cycle for Artificial Intelligence, 2018” Gartner (July 24, 2018). https://www.gartner.com/doc/3883863/hype-cycle-artificial-intelligence-/
- 6
- Sennaar, K. “Machine Learning for Medical Diagnosis – 4 Current Applications” Embro (March 5, 2019). https://emerj.com/ai-sector-overviews/machine-learning-medical-diagnostics-4-current-applications/
- 7
- Chung, E. “AI must turn focus to safety, Stephen Hawking and other researchers say”. Canadian Broadcasting Corporation (January 13, 2015).
- 8
- Bostrom, N. “Existential Risks: Analyzing Human Extinction Scenarios and Related Hazards” Journal of Evolution and Technology, Vol 9, No. 1 (2002).
- 9
- McMillan, Robert “AI Has Arrived, and That Really Worries the World’s Brightest Minds”. Wired (January 16, 2015).
- 10
- Moravac H. “When will computer hardware match the human brain?” Journal of Evolution and Technology, Vol 1, (1998).
- 11
- Kurzweil, R. “The Singularity is Near”, New York: Viking Press (2005).
- 12
- Shulman, C. and Bostrom, N. “How Hard is Artificial Intelligence? Evolutionary Arguments and Selection Effects” Journal of Consciousness Studies, Vol 19, No. 7–8, 103–130 (2012).
- 13
- Chalmers, D. “The Singularity: A Philosophical Analysis” Journal of Consciousness Studies, 17 (9–10), pp 7–65 (2010).
- 14
- Moravac, H. Estimates of brain computation based on retinal processing of information in Mind Children, Harvard University Press, pages 57 and 163 (1988).
- 15
- Merkle, R.C. “Energy Limits to the Computational Power of the Human Brain” Foresight Update, No. 6, (August 1989). http://www.merkle.com/brainLimits.html
- 16
- Grace, K., Salvatier, J., Dafoe, A., Zhang, B. and Evans, O. 2017 When Will AI Exceed Human Performance? Evidence from AI Experts” arXiv.org>cs>arXiv:1705.08807 Journal of Artificial Intelligence Research (2017).
- 17
- Grace, K. and Christiano, P. “Brain Performance in TEPS”, AI Impacts (June 6, 2015). https://aiimpacts.org/brain-performance-in-teps/
- 18
- Hsu, J. “Estimate: Human Brain 30 Times Faster than Best Supercomputers” IEEE Spectrum (August 25, 2015). https://spectrum.ieee.org/tech-talk/computing/networks/estimate-human-brain-30-times-faster-than-bestsupercomputers
- 19
- Top500 “Top 500 List of Supercomputers” Top500.org (2019).
- 20
- Dongarra, J. J., Luszczek, P. and Petitet, A. “The LINPACK Benchmark: Past, Present and Future” (2001). http://www.netlib.org/utk/people/JackDongarra/PAPERS/hpl.pdf
- 21
- Hilbert, M. and Lopez, P. “How to Measure the World’s Technological Capacity to Communicate, Store, and Compute Information Part I: Results and Scope” International Journal of Communication, Vol 6 (2015).
- 22
- Grace, K. “Global Computing Capacity” AI Impacts (February 16, 2016).
- 23
- Basser PJ, Pajevic S, Pierpaoli C, Duda J, and Aldroubi A. “In vivo fiber tractography using DT-MRI data”. Magnetic Resonance in Medicine. 44 (4): 625–32 (October 2000).
- 24
- Alivisatos, A. Paul; Chun, Miyoung; Church, George M.; Greenspan, Ralph J.; Roukes, Michael L.; Yuste, Rafael (June 2012). “The Brain Activity Map Project and the Challenge of Functional Connectomics” Neuron. 74 (6): 970– 974 (February 22, 2013).
- 25
- Huang, T-H., Niesman, P., Arasu, D., Lee, D., De La Cruz, A., Callejas, a., Hong, E. J. and Lois, C. “Tracing neuronal circuits in transgenic animals by transneuronal control of transcription (TRACT)” eLife, 6. Art. No e32027. ISSN 2050–084X (2017).
- 26
- Bargmann, C., Newsome, B. (co-chairs) “Brain Research through Advancing Innovative Neurotechnologies (BRAIN) Working Group. National Institutes of Health (April 24, 2013).
- 27
- Amunts, K., Ebell, C., Muller, J., Telefont, M., Knoll, A., and Lippert, T. “The Human Brain Project: Creating a European Research Infrastructure to Decode the Human Brain”. Neuron. 92 (3): 574–581 (2016).
- 28
- Baeck, T., Fogel, D. B. and Muchalewicz, T. “Handbook of Evolutionary Computation” CRC Press, Boca Raton (1997).
- 29
- Coello, C. A. C. “A Comprehensive Survey of Evolutionary-Based Multiobjective Optimization Techniques” Knowledge and Information Systems, V 1, Is 3, 269–308 (1999).
- 30
- Mukherjee, A. and Siewiorek, D. P. “Measuring Software Dependability by Robustness Benchmarking” IEEE Transactions on Software, V 23, Is 6 (1997).
- 31
- Terrile, R. J. and Guillaume, A. “Evolutionary Computation for the Identification of Emergent Behavior in Autonomous Systems.” IEEE Aerospace Conference Proceedings, Big Sky, MT (March 2009).
- 32
- Terrile, R. J., Adami, C., Aghazarian, H., Chau, S. N., Dang, V. T., Ferguson, M. I., Fink, W., Huntsberger, T. L., Klimeck, G., Kordon, M. A., Lee, S., von Allmen, P. A. and Xu, J. (2005) “Evolutionary Computation Technologies for Space Systems” IEEE Aerospace Conference Proceedings, Big Sky, MT (March 2005).
- 33
- Miley, J. “11 Times AI Beat Humans at Games, Art, Law and Everything in Between” Intereseting Engineering,
(March 12, 2018).
- 34
- Honderich T “Consciousness”. In. The Oxford companion to philosophy. Oxford University Press (1995).
- 35
- Turing, A. “Computing Machinery and Intelligence”, Mind, LIX (236): 433–460 (October 1950).
- 36
- Hruska, J. “Did Google’s Duplex AI Demo Just Pass the Turing Test?” Extreme Tech (May 9, 2018).
- 37
- Gallup, GG Jr. “Chimpanzees: Self recognition”. Science. 167(3914): 86–87 (1970).
- 38
- Kwiatkowski, R. and Lipson, H. “Task-agnostic self-modeling manchines” Science Robotics, V 4, Issue 26, (January 30, 2018).
- 39
- Kwiatkowski, R. and Lipson, H. “Task Agnostic Self-Modeling Machines” Columbia Engineering (January 25, 2019). https://www.youtube.com/watch?v=4dp_iiESLo8&feature=youtu.be
- 40
- Argonov, V. “Experimental Methods for Unraveling the Mind-body Problem: The Phenomenal Judgment Approach”. Journal of Mind and Behavior. 35: 51–70 (2014).
- 41
- Demertzi, A., Tagliazucchi, E., Dehaene, S., Deco, G., Barttfeld, P., Raimondo, F., Martial, C., Fernández-Espejo, D., Rohaut, B., Voss, H. U., Schiff, N. D., Owen, A. M., Laureys, S., Naccache, L. and Sitt, J. D. “Human consciousness is supported by dynamic complex patterns of brain signal coordination” Science Advances, v 5, 2, (February 6, 2019).
- 42
- McDonald, I. River of Gods, Simon and Schuster, United Kingdom, Chapter 4 p 42 (2006).
- 43
- Bostrum, N. “Are You Living In a Computer Simulation?” Philosophical Quarterly, V 53, No. 221, pp 243–255 (2001).
- 44
- Wigner, E. and Margenau, H. “Remarks on the Mind Body Question, in Symmetries and Reflections, Scientific Essays” American Journal of Physics, 35, 1169–1170 (1967).
- 45
- Tegmark, M. “Is “the Theory of Everything” Merely the Ultimate Ensemble Theory?”. Annals of Physics. 270 (1): 1–51 (November 1998).
- 46
- Tegmark, M. “Our Mathematical Universe”, Knopf (2014).
- 47
- Bostrom, N. and Yudkowsky, E. “The Ethics of Artificial Intelligence” Cambridge Handbook of Artificial Intelligence, Cambridge University Press (2011).
- 48
- Bostrom, N. “The Superintelligent Will: Motivation and Instrumental Rationality in Advanced Artificial Agents” Minds and Machines (2012). https://nickbostrom.com/superintelligentwill.pdf